Social Media Snooping
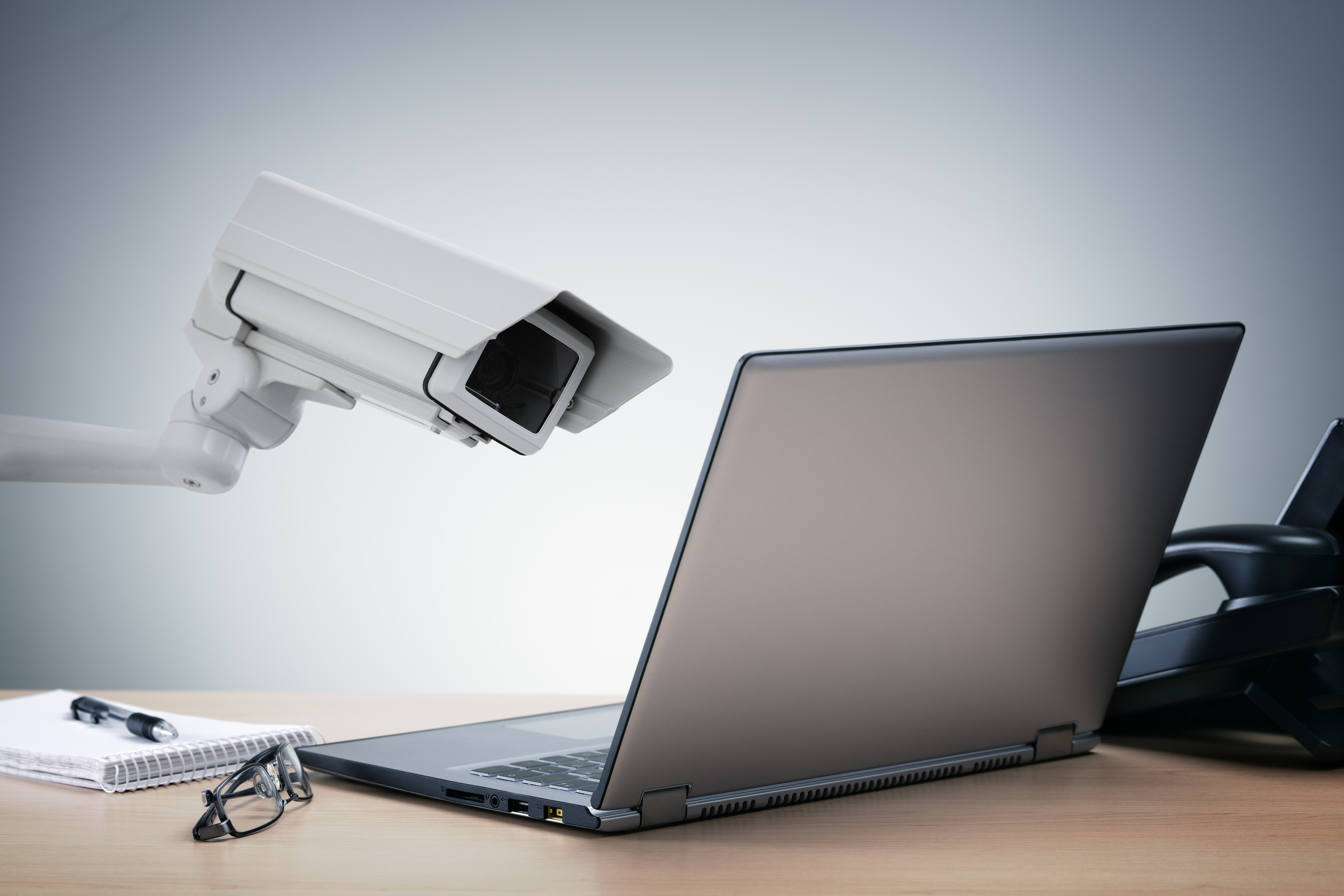
Here we go again. More taxpayer funded “research” to look at what average, everyday people are saying on Twitter. I’ve written about this type of research before, and no doubt I’ll end up writing about it again, and again, and again ad infinitum.
The supposed aim of this spectacular pile of fetid, festering, dingo kidneys is to try and automatically classify Twitter users who tweet about e-cigarettes into “distinct categories”.
I guess this lot were bored or had a stack of cash floating around that was about to be nabbed by something worthwhile, or they had another study idea that needed a cash injection so they needed to waste cash to get more. Typical tobacco control “research” thinking troughing.
Methods
We collected approximately 11.5 million e-cigarette–related tweets posted between November 2014 and October 2016 and obtained a random sample of Twitter users who tweeted about e-cigarettes. Trained human coders examined the handles’ profiles and manually categorized each as one of the following user types: individual (n=2168), vaper enthusiast (n=334), informed agency (n=622), marketer (n=752), and spammer (n=1021). Next, the Twitter metadata as well as a sample of tweets for each labeled user were gathered, and features that reflect users’ metadata and tweeting behavior were analyzed. Finally, multiple machine learning algorithms were tested to identify a model with the best performance in classifying user types.
Bless. These people really believe that a vaper on Twitter does nothing but tweet about vaping.
Twitter’s pervasiveness makes it a convenient tool for e-cigarette manufacturers, enthusiasts, and advocates to promote e-cigarettes actively to a wide audience.
Look, that’s just not how Twitter works. At all. I follow 1,300 accounts on Twitter, which isn’t a particularly big number compared to some users. I also have 1,806 followers (you crazy, crazy people!). Whenever I tweet or retweet something, only those 1,806 will see that - initially. Should some among my followers decide to retweet my stuff, then **their followers** will see it, even if some of those followers don’t follow me. Follow me? (pun totally intended, obvs)
Naturally, there’s going to be some overlap between accounts. I know that a number of folks that follow me, also follow people I follow. I also have a number of hashtag searches I keep an eye on which enables me to see accounts tweeting about that hashtag (though sometimes there’s utter bilge in there) that I don’t follow.
See, that’s the thing about Twitter. It’s a wide-open platform, but the majority of users follow other like-minded users, thereby creating secular “communities” among the estimated 316 million active Twitter users. Of course, when researchers want to analyse stuff from Twitter, they get access to the API so they can see everything.
Using Twitter’s enterprise application programming interface (API) platform, Gnip, we collected e-cigarette–related tweets posted between November 2014 and October 2016. A comprehensive search syntax was developed with 158 keywords, including terms such as ecig, vape, and ejuice, as well as popular e-cigarette brands and hashtags, which resulted in approximately 11.5 million e-cigarette–related tweets from 2.6 million unique users.
They used specific keywords, including brands. Rather strangely, the authors don’t list the actual keywords they used (as others have extensively done) so I’m left sucking wind at what keywords (other than the examples provided) they actually used to get 11.5 million tweets and 2.6 million users. I would suspect that many of the keywords used in the search are likely to be meaningless and could match any number of candidates.
Once they had the data, it needed to be reviewed, and this is where it gets a bit creepy.
Six coders were trained using the protocol and practice data to classify the user types manually. For each user, the coders reviewed the user’s profile page on Twitter, which included a profile description and a sample of recent tweets on their timeline, which may have included e-cigarette and non-e-cigarette topics.
Yep. The coders visited every Twitter profile page of the 2.6 million matched users. Read the bio and examined the most recent tweets to classify that person. Very Big Brother. Each account was then classified as one of five categories:
Out of the 2.6 million users, 4897 were manually classified into one of those categories. Amusingly, individual held the greatest number which would put pay to the incessant shrieking of “Big Tobacco shill!”, or so you would think. But then, since when did evidence ever matter to tobacco control?
Onwards to additional data collection then. Behaviour. Oh yes, these folks analysed additional twitter metadata to determine the behaviour of the user:
It was hypothesized that tweeting behaviors would vary across different user types (eg, individuals are likely to tweet about more diverse topics than marketers). Studies have shown that linguistic content of social media posts is particularly useful because it illustrates the topics of interest to a user and provides information about their lexical usage that may be predictive of certain user types
Which means they looked at the account followers/followed ratio, profile image (creeped out yet?), whether geo-location was enabled, total retweet count, verified (or not), counting hashtags in a tweet, link count, number of times a tweet has been “liked” as well as retweeted. The list is quite extensive and a little unnerving.
After compiling that list, the researchers looked at the 200 most recent tweets from the 4897 selected users - don’t you feel special?
Naturally, the authors had to check to see if their “automated” machine learning was in any way accurate, which meant further review:
To further examine variations in the predictive performance across user types, a confusion matrix illustrating predicted and actual user types was generated. Figure 2 shows the distribution of predicted user types on the horizontal axis and actual user types from the manual coding on the vertical axis. To aid in interpretation, the predicted sample proportion for each user type is shaded from light (low proportion) to dark (high proportion). Darker shading in the cells along the diagonal indicates correct classification, whereas darker shading elsewhere indicates misclassification.
Rather unnervingly, it seems that the machine model is rather accurate, at least as far as normal, everyday individuals are concerned. Out of the 325 users manually coded as individuals, 300 of them were correctly predicted by the machine to be individuals.
Picking out enthusiasts, however, is a different story. Only 20 of the 50 enthusiasts were correctly predicted, while 22 were misclassified as marketers - which explains why so many of us out in the twitterverse are misconstrued as such.
As mentioned earlier, twitter follows & followers, along with what you tweet about passively creates distinct clusters, a fact that is highlighted in the following image:
Aside from looking rather cool, it is quite clear that individuals tend to be rather isolated from the “informed agency” and marketer type accounts, while enthusiasts tend to mix things up a bit.
Vaper enthusiasts also comprise a distinct cluster, but there appears to be a substantial overlap between vaper enthusiast and marketer clusters.
A not so subtle way of saying enthusiasts are part marketers, or to use the antipodean pensioners’ preferred term, shills for big tobacco (or big vapour).
By the way, “informed agency” is treated as a “trusted source” in this paper. Which will include accounts like @FCTCOfficial, @WHO, and everyone’s favourite @FDATobacco, among others. Trusted sources my arse.
The point of this exercise?
FDA has the authority to regulate claims made by e-cigarette companies and will need to monitor e-cigarette brand social media handles to ensure that they are being compliant with regulatory policies (eg, not making cessation claims, posting warning statements about the harmful effects of nicotine). In contrast, FDA cannot regulate claims made by vaper enthusiasts because they are individuals and not companies selling e-cigarette products. Therefore, distinguishing vaper enthusiasts from marketers is critical to informing FDA compliance and enforcement efforts.
Ah. Regulation. Natch.
Being able to distinguish vaper enthusiasts from marketers is also important with regard to public health education efforts because vaper enthusiasts have been known to undermine e?cigarette education campaigns. For example, when the California Department of Public Health launched its Still Blowing Smoke campaign to educate consumers about the potential harmful effects of e-cigarette use, vaper advocates launched a countercampaign (Not Blowing Smoke). By using both hashtags and creating new accounts, the countercampaign attacked the credibility of messages of the California Department of Public Health and effectively controlled the messaging on social media.
Of course, the Ministry of Truth. Then of course, there’s Big Brother:
We would argue that classifying marketers and vaper enthusiasts separately is important for informing e-cigarette surveillance, regulatory, and education efforts.
“Know thy enemy”
Conclusion
In conclusion, this study provides a method for classifying five different types of users who tweet about e-cigarettes. Our model achieved high levels of classification performance for most groups; examining tweeting behavior was critical in improving the model performance. The results of our approach can help identify groups engaged in conversations about e-cigarettes online to help inform public health surveillance, education, and regulatory efforts. Future studies should examine approaches to improve the classification of certain user groups that were more challenging to predict (eg, vaper enthusiasts).
I sense a pattern emerging from this social science. By being able to identify (either manually or automatically) who vapers are, it’ll make it easier to generate a list of accounts for the ivory tower residents to pre-emptively block, and shut down any kind of debate (which they do already, this’ll just make it easier).
As far as I know, no other product/hobby/interest has this much social media investigation.
Are they scared?
(image credit Brian A Jackson/shutterstock.com)